Top 3 Questions About Deep Learning: What Is Deep Learning? How Does Deep Learning Work? Why Need Deep Learning? These are some of the most commonly asked questions about deep learning.
Deep learning applications are employed in various sectors, ranging from autonomous driving to medical equipment. It is the key to voice control on telephones, television, and tablet. Deep learning has charmed a lot of care. It is accomplishing previously unthinkable achievements.
In this article, we’ll explore the top 3 questions about deep learning in more detail, providing you with valuable insights from leading experts in the field. It is hard to understand deep learning logic, but we will try to explain deep learning to a child. If you’re curious to learn more detail about deep learning and its applications, you’re in the right place. So, without further ado, let’s dive into the fascinating world of deep learning and discover why it is poised to become the driving force behind the next wave of technological innovation.
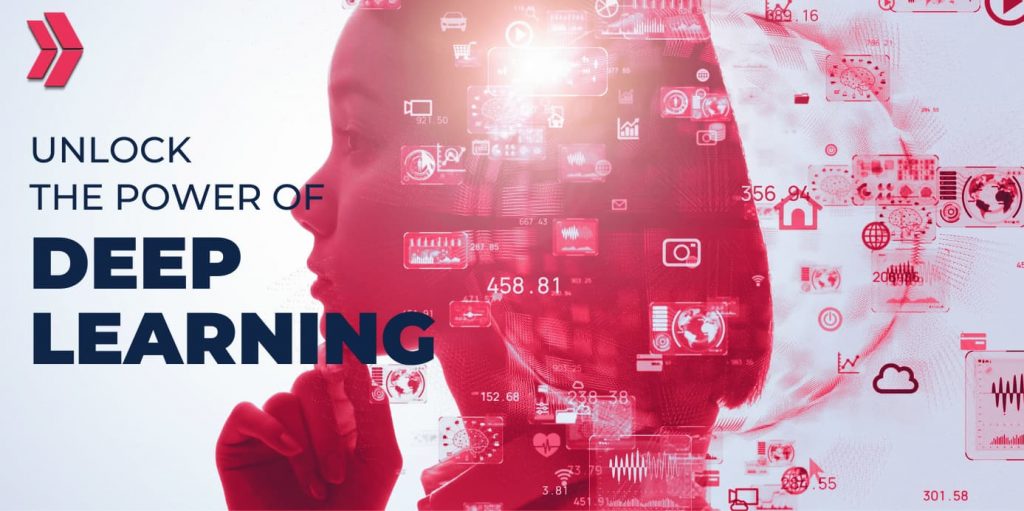
What is Deep Learning?
Deep learning is a subfield of machine learning that focuses on teaching computers to learn and recognize patterns by mimicking the way the human brain processes information. Deep learning is concerned with artificial neural networks. Artificial neural networks are algorithms that are intended to imitate the way human beings consider and understand.
Deep learning learns automatically from examples and improves functions by examining these algorithms via vast volumes of data. At least three neural network layers are required for deep learning. For example, the deep learning behind driverless cars uses voice control in consumer devices like phones, tablets, TVs, and hands-free speakers.
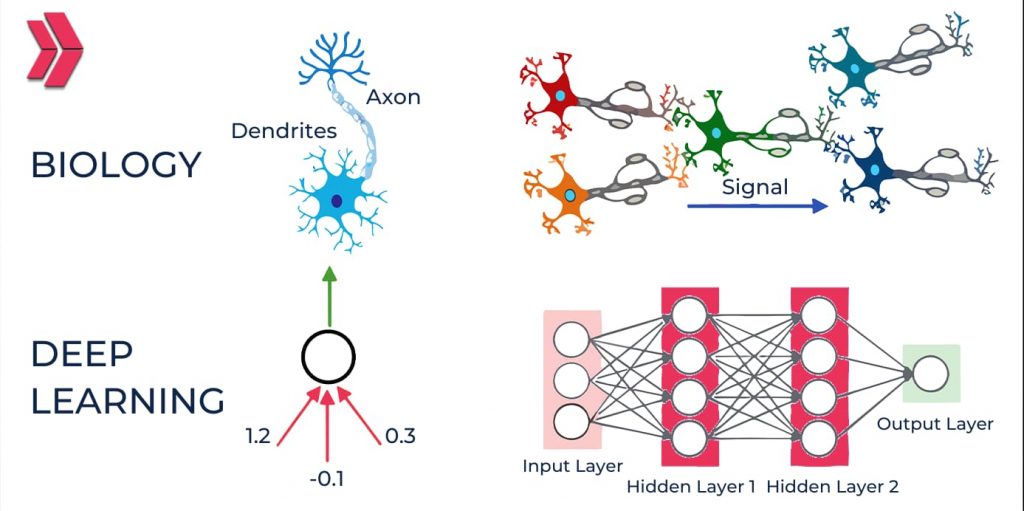
How Does Deep Learning Work?
Deep learning works by training artificial neural networks to learn and make predictions or decisions. Understanding how deep learning works is crucial. The human brain comprises billions of neurons. Similarly, neural networks are also made of nodes. One neuron in the human brain gets millions of impulses from neighboring neurons. Nodes in layers are connected to nodes in neighboring layers, with each layer improving and refining the prediction or categorization.
The amount of layers in the network influences its depth. Signs act betwixt nodes in the artificial neural network, and weights are allocated to those nodes. A heavier-weighted node will have a greater influence on the following sequence of nodes. The final layer produces an output by combining the weighted inputs.
The input and output layers of a deep neural network are the two visible layers of a deep neural network. The input layer receives data for processing, while the output layer performs the final prediction or classification. For example, if the deep learning algorithm fails to detect a pattern, it changes its weights and tries again until it has learned all the data’s patterns.
There are two kinds of propagation while working on deep learning: forward propagation and backpropagation. Forward propagation describes how computations flow through a network. Backpropagation measures forecasting errors using techniques like gradient descent, modifies the weights and biases of the function by iterating back through the layers, and trains the model.
While working with deep learning, some well-known models, like Recurrent Neural Networks (RNN) and Convolutional Neural Networks (CNN), can be used.
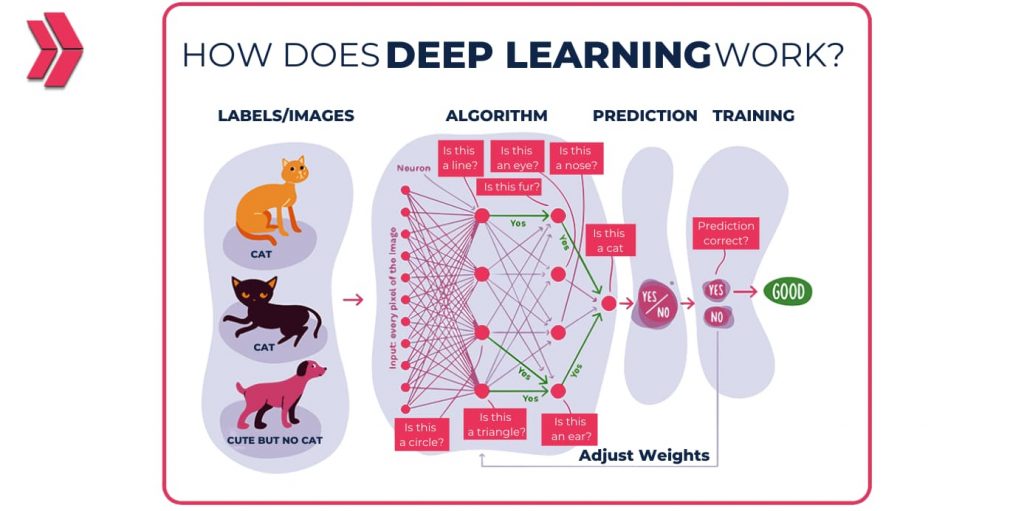
Why Do We Need Deep Learning?
Deep learning is essential for image classification and natural language processing. Deep learning is required because:
- Deep learning algorithms can independently extract features from data.
- From the given tasks, deep learning may learn complex or hidden patterns.
- Algorithms for deep learning can process enormous amounts of data.
- Through deep learning, complex interactions between a large number of interdependent factors can be understood.
- Deep learning may be used to examine data from a variety of sources, such as audio, video, pictures, numbers, and documents.
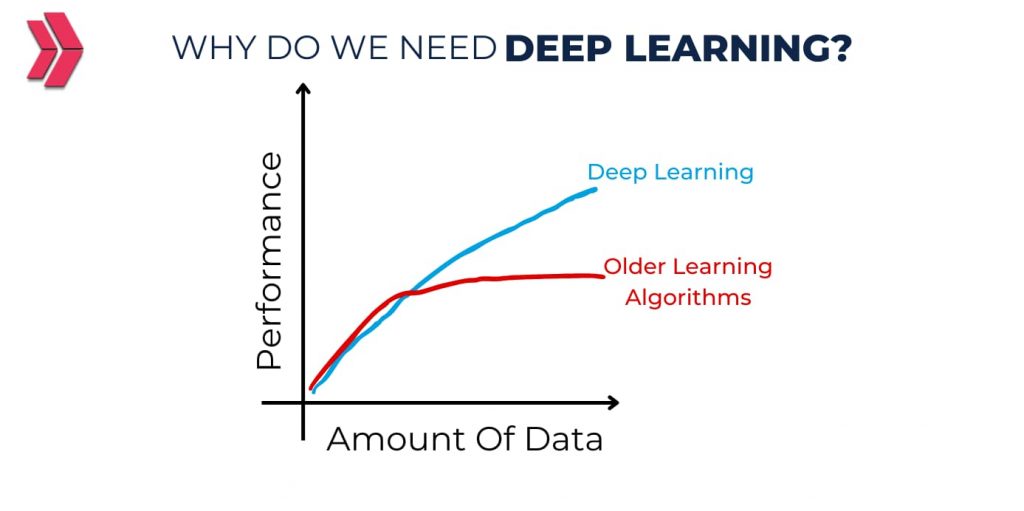
What’s the Difference Between Machine Learning and Deep Learning?
Machine learning and deep learning are two subsets of artificial intelligence (AI) that share similarities but also have distinct differences.
Machine learning algorithms create predictions using structured, labeled data, which is usually pre-processed to be organized in a structured manner. If you want to understand more about machine learning, check out the article What Is Machine Learning?
Deep learning is a subset of machine learning that reduces part of the data pre-processing required by machine learning. It automates feature extraction, reducing the need on human specialists.
Here are the general and the most important differences between deep learning and machine learning:
- One of the first differences between machine learning and deep learning is that deep learning algorithms are layered in ascending order of complexity and abstraction and take significantly longer to train than machine learning algorithms. However, with more training datasets, deep learning models outperform.
- Features and a classifier are manually chosen in machine learning to organize images. The phases of feature extraction and modeling are automated with deep learning.
- In contrast to linear machine learning algorithms, the abstraction and complexity of deep learning algorithms are stacked in a hierarchy.
- A domain expert is needed to determine the most used features in machine learning. On the other hand, deep learning gradually comprehends characteristics, negating the requirement for domain knowledge.
- In contrast to machine learning, which relies on more basic concepts, deep learning uses artificial neural networks designed to imitate how people consider and understand.
- Machine learning does not need high-end computers and high-performance GPUs like deep learning.
- After training with large data sets, machine learning algorithms’ performance tends to plateau, and then diminishing returns kick in. Deep learning models, on the other hand, perform better as the amount of training datasets increases.
You can read the article named Deep Learning Vs. Machine Learning explained to understand more about machine learning and deep learning.
How to Create and Train Deep Learning Models?
The following are the most popular applications to create and train for deep learning models:
From Scratch Training
Gathering labeled data before building a deep network is beneficial for new applications and apps but requires a large quantity of data and can take days or weeks to train.
Transfer Learning
Transfer learning involves modifying a pre-trained model to create new jobs with specific categorization skills, reducing calculation time to minutes or hours.
Feature Extraction
Feature extraction machine learning models can remove characteristics from the network and use them as input.
What are the Deep Learning Applications?
Deep learning has found applications in various domains and has revolutionized several fields. Deep learning applications are integrated into everyday life, but users are often unaware of their processing. Here are some prominent applications of deep learning:
Self-Driving Vehicles
Deep learning is being used to recognize and identify pedestrians, reducing accidents.
Medical Research
Deep learning is being used by cancer researchers to automatically identify cancer cells.
Defense
Deep learning is used in defense to detect safe and risky zones for troops.
Aerospace
Deep learning is used in aerospace to distinguish objects detected by satellites that target areas of interest.
Industrial Automation
Deep learning enhances employee protection by automatically recognizing when a worker or object approaches a machine.
Financial Services
Financial institutions use predictive analytics to manage credit and investment portfolios.
Text Generation
Machines use a model to automatically write a new text that matches the original text’s grammar, spelling, grammar, and style.
Computer Vision
Deep learning improves computer vision, allowing for object identification, picture categorization, restoration, segmentation, self-driving vehicles, drones, and biometric operations.
Natural Language Processing (NLP)
Text classification, sentiment analysis, translation, speech recognition, and other applications use NLP algorithms to analyze and interpret human language inputs.
What are Deep Learning Jobs?
Deep learning has gained significant popularity, leading to a high demand for professionals with expertise in this field. Data scientist is the general job name of this sector. The following are the top four deep learning jobs to consider:
Deep Learning Engineer
Deep learning engineers create complex neural models and build relevant ML algorithms with prior knowledge of software engineering best practices.
Deep Learning Scientist
Deep learning scientists design, build, configure, and optimize many different applications for deep neural networks. The improvement of deep learning and machine learning models is the responsibility of deep learning scientists.
NLP Scientist
NLP scientists create deep learning models and apps to understand and translate speech patterns. Their primary objective is to develop systems capable of understanding human languages as people do.
Image Processing Engineer
Image processing engineers use ML approaches to evaluate data and build algorithms, to visualize findings and convey results.
What Are the Advantages of Deep Learning?
The following are the advantages of deep learning:
- Take on difficult problems such as audio processing in Amazon Echo, image recognition, and so on.
- Reduce the requirement for feature extraction.
- Automated tasks that use Keras and TensorFlow to provide predictions in less time.
- Parallel computation is possible, which reduces overhead.
- Models may be trained on massive amounts of data, and the model improves as more data is added.
- By training incessantly, it is possible to get higher-quality predictions than humans.
- Unstructured data works well, including video clips, documents, sensors, and webcam data, among other things.
What Are the Limitations of Deep Learning?
While deep learning has shown remarkable capabilities in various domains, it also has certain limitations that should be considered. We can classify the deep learning limitations like the below. The written below are very important for the deep learning interview questions and deep learning questions you may encounter in your career. Here are some of the limitations of deep learning:
Data Dependency
These are about data dependency of deep learning:
- More powerful and precise models require more data.
- Dependencies between production-consumption links indicate that B must utilize data produced by A and C must use data produced by B.
- Deep learning models only learn through observation, not training data.
- The convergence of deep learning models could be too fast or too slow, producing less-than-ideal results.
Overfitting
These are about the overfitting of deep learning:
- A model will reproduce the same biases in its forecasts if it was trained on biased data.
- Preventative measures such as early stopping and reducing model complexity can help reduce overfitting.
- Underfitting occurs when the model has not been trained for a suitable period of time or when the input variables are not statistically meaningful enough.
- Underfit models have greater bias and lower variation in their predictions.
- The model’s bias lowers as it learns, but its variance grows as it gets overfit.
Interpretability
These are the ethical implications of deep learning:
- Testing approaches differ greatly, making assessing performance in real-world applications problematic.
- It learns on its own, and it’s also difficult to monitor a system’s progress over time.
- It’s difficult to express in words and is not completely understood.
- Deep learning cannot handle complex applications, such as programming and scientific methods.
- They are utterly incapable of long-term planning and data processing akin to an algorithm.
Ethical Considerations
These are the ethical considerations of deep learning:
- Justice and equity.
- Liberty, autonomy.
- Transparency in privacy.
- Trust.
- Beneficence.
- Responsibility.
- Patient security and faith in cyber security.
- Solidarity.
- Sustainability.
- Dignity.
- Conflicts.
Future of Deep Learning
The future of deep learning holds immense potential and is likely to witness significant advancements and transformations. Deep learning is still in its early phases, and it has a lot of room to expand. Deep learning will become more frequently employed in the future and will have a huge influence on the globe. Deep learning will have the following effects on the world:
Emerging Trends
Here are some of the trends of deep learning;
- Self-supervised learning.
- Neuroscience-based deep learning.
- System 2 deep learning.
- Deep learning + symbolic AI.
- Containerized workloads.
- AI and model training.
- Hybrid Model Integration.
- The Vision Transformer.
- High-Performance NLP Models.
- Use of Edge Intelligence.
- Vision Transformers.
- Multimodal multitasking transformers.
- General adversarial networks (GAN).
Potential Deep Learning Applications in the Future
Deep learning has several applications in a range of industries. Let’s take a look at some of the most notable deep-learning applications:
Image Recognition
Deep learning may be used to detect items in photos automatically. This is helpful for security, automotive, and medical applications, among others.
Detecting Objects
Deep learning may be used to detect items in photos or movies. This is important for security, surveillance, and industrial inspection jobs.
Deep Learning for Video Analysis
Video data analysis may make use of deep learning. This is useful for facial identification, behavior analysis, and sports analytics.
Natural Language Processing
Deep learning analyzes and understands natural language data. Chatbots, machine translation, and text classification can all benefit from this.
Medical Care
Deep learning has enabled medical picture analysis, diagnosis, and treatment.
Stock Evaluation
Quantitative financial analysts use metrics such as return on a stock, P/E ratio, return on money, bonus, return on capital used, profit per worker, and the total amount of money to train deep learning layers.
Detection of Fraud
Deep learning can detect fraudulent transactions on the internet, saving financial firms billions of dollars by identifying outliers.
News Evaluation
The government is restricting the dissemination of fake news and its source, but there are limitations, such as the inability to verify the veracity of data.
Autonomous Vehicles
Deep learning is used in self-driving cars to evaluate data gathered from sensors, open cameras, and other sources.
Impact on Society
Deep learning, as a powerful and versatile technology, has the potential to significantly impact various aspects of society. Here are some impacts of deep learning on society:
- The period of time between employment disappearing and new ones appearing.
- AI automation of tasks raises ethical implications due to the societal effects of job losses among humans. Hence, job displacement will have a significant negative impact on the lowest 90% of the global population, notably the bottom 50% in terms of income or education.
- By using algorithms, deep learning can decrease human error and improve weather forecasting accuracy.
- Robots can take on dangerous tasks like bomb disposal, underwater exploration at great depths, and coal and oil extraction in place of people.
- Machines can operate continuously, without pauses, for a wide range of requests and problems.
- When repetitive chores are automated, human beings can engage in more creative endeavors, like document verification for loan applications.
- AI-based technology can aid people in the solution of challenging issues, such as the prediction of breast cancer.
Deep Learning With Clarusway
As deep learning technology is growing, and as deep learning technology progresses, more such intriguing deep learning employment options will arise. Never before has there been a better moment to participate in this revolutionary technology. If you would like further information about deep learning, you can enroll in our machine learning course.
The machine learning course is a 13-week program comprising 135+ hours of in-class sessions and 54+ hours of Career Management Services (CMS). Life coaching, resume writing, LinkedIn training, and interview preparation support are among our specialty CMS activities for the Machine Learning program. Besides the curriculum, you can practice what you have learned through hands-on activities + 10 projects + 3 Capstone Projects.
Are you ready to give your career a boost? Enroll Now!
Last Updated on August 29, 2023